Tech
The Future of AI: Top Applications of Multimodal Models
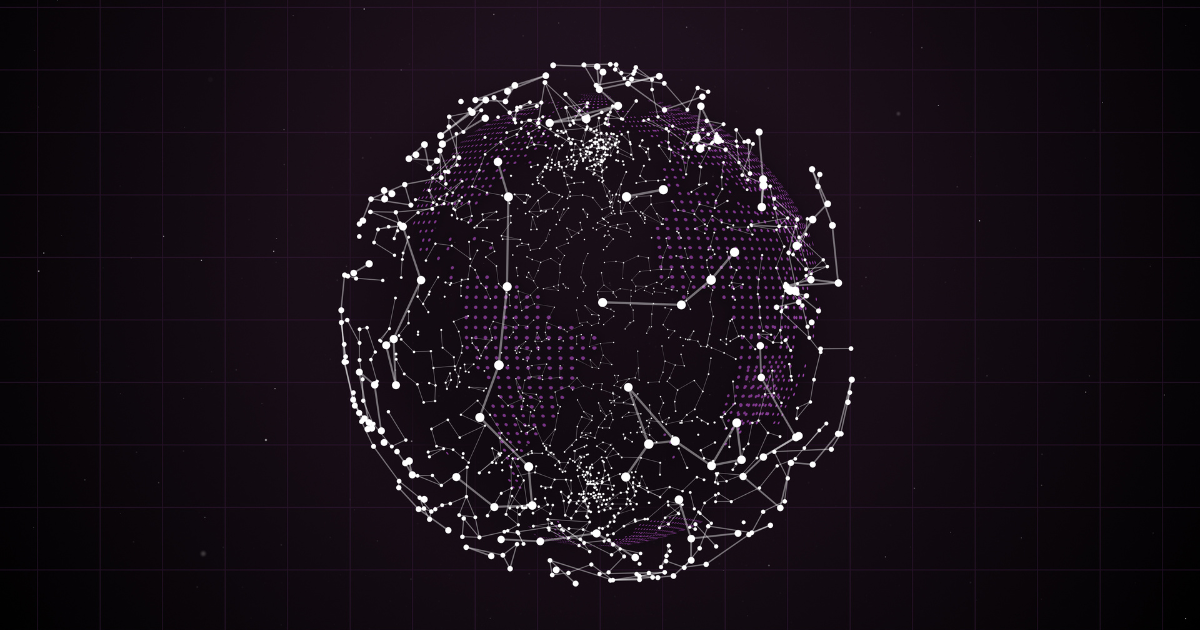
The evolution of AI is gaining momentum, and one of its key developments is multimodal models. Forecasts for the coming years predict rapid growth in the global AI market based on these advanced models. According to KBV research,this market is expected to reach an impressive $8.4 billion by 2030, growing at an incredible 32.3% CAGR.
This fascinating phenomenon confirms the important role of multimodal models. Further exploration is worth the effort. We can find specific applications and benefits that these advanced technologies bring to various areas of life.
Multimodal – the future of AI
The launch of Chat GPT 3.5Â was a milestone in AI development. Although this tool is far from being truly human intelligence, it is a major step towards creating its digital counterpart. GPT 3.5 generates text, summarizes it, and answers queries. This means that it is limited to one modality – text.
The real revolution turned out to be the GPT 4 by enhancing multimodality. This powerful, versatile system not only generates text but also handles a variety of visual and audio information.
Thereby, multimodal AI combines information from vision, sound, and text. This innovative approach enables AI systems to analyze the world more comprehensively.
Moreover, it brings them closer to human perception by using different modalities at the same time. As a result, multimodality is the future of AI. Unlock the full potential of multimodal models with expert AI consulting services, tailored to help you leverage multimodal models for advanced, real-world applications.
The importance of multimodal models
Multimodal models are a key step in the development of AI, combining diverse types of data such as text, images, audio, and video. They consist of three main components:
- Unimodal encoders, which process data from each modality independently
- A fusion network, which integrates the extracted features into a coherent representation
- A classifier generating predictions from the combined data
The benefits of multimodal models are significant. Their ability to process multiple data sources simultaneously translates into increased forecast accuracy. As they can analyze and integrate data from different sources, they can solve complex problems. The flexibility and versatility of these models allow them to deal effectively with different types of input data. In addition, their robustness comes from the use of multiple data sources, which makes them reliable even when one modality is missing or damaged.
Multimodal applications
Multimodal models are finding wide applications, revolutionizing many fields. Here are some notable use cases.
HEALTHCARE
Multimodal models improve diagnostic accuracy by integrating a variety of data sources. These advanced systems enable accurate assessment of patient conditions by simultaneously analyzing:
- Medical imaging
- Patient history
- Laboratory tests
- Genomic data
This synergy of information allows for more precise diagnoses and prediction of patient outcomes. Hence, multimodal AI becomes not only a diagnostic tool with increased accuracy. It also supports the development of patient-centered healthcare.
SECURITY
Multimodal models are crucial in the security field. They introduce new possibilities for analysis and monitoring. Integrating different data sources enables a more comprehensive approach to security issues.
In the context of video surveillance, these models are able to analyze not only images but also interpret sounds and textual data. It results in a more comprehensive assessment of the situation.
In the area of cyber security, multimodal analysis of text and audio data can effectively identify potential threats. In addition, these models can enable rapid response to emergency situations.
AUTOMOTIVE INDUSTRY
Autonomous vehicles are our future, and they will shape it. They are the ones that can significantly increase road safety by eliminating the main cause of accidents – human error.
It is thanks to AI that the first fully autonomous vehicles are already moving on some roads. Another multimodal application of the models is in the automotive industry.
These advanced models enable complex perception of the environment. They process and interpret data from a variety of sensors, such as cameras, lidar, radar and ultrasonic sensors. As a result, autonomous vehicles can better understand their surroundings. As a result, multimodal models support safe navigation and enable informed decision-making.
ASSISTIVE TECHNOLOGY
Assistive technology for people with disabilities also uses multimodal models. These advanced systems integrate different forms of communication, enabling more effective interaction. Multimodal models analyze both speech and images to better understand user intent. In this way, multimodal models improve the quality of life for people with disabilities.
IMAGE CAPTIONING
Image Captioning is a technology based on multimodal models to generate image descriptions. These advanced models integrate visual and textual data to understand the context of an image. By analyzing images, multimodal models extract key information and then generate the appropriate captioning. As a result, we can better understand visual content.
Conclusion
Multimodal models are a key step in the evolution of AI, allowing for the integration of textual, visual, and audio data. Applications of these models include areas such as:
- Healthcare
- Security
- Automotive industry
- Assistive technology
- Image captioning
They can process multiple data sources at once, so they are very effective at analyzing and solving complex problems. Multimodal model applications are a key step forward, enabling more advanced and effective use of AI.
SEE ALSO: Power Of Microsoft PowerPoint: 5 Uncommon Features For Enhanced Presentations